Reach your Academic Goals hassle-free. Get Dissertation Editing help at the click of a button
Get Online Dissertation Editing, Formatting, and Proofreading Help From Best Editors Instantly!!
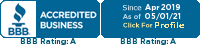
Expert Dissertation Help Services
In statistics and research design, the term covariate often arises, especially in fields involving experimental studies, regression analysis, and various forms of modeling. Covariates are variables that are related to other variables of interest and have a significant impact on the interpretation of research results. Understanding covariates is essential for accurate statistical modeling and obtaining valid results. In this article, we’ll explore what covariates are, their different types, and their applications in various fields of study, as well as how to incorporate them correctly in statistical and data analyses.
What is a Covariate?
A covariate is an independent variable that may not be the primary focus of the study but can influence the dependent variable or outcome of interest. These variables are generally controlled for, measured, or accounted for in the analysis to reduce bias and improve the accuracy of the results. By including covariates in the analysis, researchers can account for potential confounding factors, thus isolating the true relationship between the main variables being studied.
In simple terms, covariates are supplementary variables that explain or contribute to the variations in the outcome but are not the primary predictors or variables of interest in the study.
Why Covariates Matter in Statistical Analysis
Covariates play a crucial role in statistical analysis for several reasons:
- Control for Confounding Variables: Covariates can control for factors that may confound the relationship between the main independent and dependent variables. This helps prevent misleading associations that could arise due to other underlying variables.
- Increase Precision: By including covariates, researchers can reduce unexplained variability, leading to more precise estimates of the effect of the independent variable on the dependent variable.
- Improve Model Fit: In regression and other models, covariates can improve model fit by explaining more of the variation in the dependent variable.
- Provide Insight into Secondary Relationships: Sometimes, understanding how covariates relate to the outcome can offer valuable insights into the research topic, even if they’re not the primary focus.
Types of Covariates
Covariates can be classified in various ways depending on their nature and role in the study. Here are the most common types:
- Continuous Covariates
These are covariates that have a continuous scale, meaning they can take on an infinite number of values within a given range. Examples include age, weight, height, temperature, and income level. Continuous covariates are often used in statistical models because they allow for nuanced, scalable adjustments.
- Categorical Covariates
Categorical covariates represent groups or categories and do not have a natural ordering. Examples include gender, race, education level, and marital status. These are typically included as dummy variables or indicators in statistical models to account for differences between groups.
- Time-Varying Covariates
Time-varying covariates change over time within the same individual or subject. For example, blood pressure measurements taken at different times during a study would be considered time-varying covariates. These are particularly common in longitudinal studies where measurements are taken repeatedly over time.
- Fixed Covariates
Fixed covariates are variables that remain constant for an individual over the course of the study. Examples might include birth date, genetic information, or baseline characteristics that do not change with time or intervention. Fixed covariates are often used in longitudinal studies where it’s important to control for individual baseline differences.
- Confounding Covariates
Confounding covariates are variables that can distort the relationship between the independent and dependent variables. For example, in a study assessing the impact of exercise on heart health, diet might be a confounding covariate because it could influence heart health independently of exercise.
- Mediator Covariates
Mediator covariates are variables that help to explain the mechanism or process through which an independent variable influences a dependent variable. For instance, in studying the effect of socioeconomic status on health outcomes, stress levels might be a mediator that explains part of this relationship.
How to Select Covariates
Selecting the right covariates is a critical part of designing a robust statistical analysis. Here are some common guidelines:
- Relevance to the Outcome: Covariates should have a logical and statistical relationship with the outcome variable.
- Avoiding Overfitting: Adding too many covariates can lead to overfitting, where the model becomes overly complex and loses its generalizability.
- Checking for Multicollinearity: Including covariates that are highly correlated with each other can lead to multicollinearity, which makes it difficult to distinguish their individual effects.
- Use of Prior Research: Drawing on prior studies can help identify covariates that have consistently shown an effect on the outcome of interest.
- Availability of Data: It’s essential that the data for chosen covariates are available, complete, and reliable.
- Avoiding Post-hoc Decisions: Selecting covariates after analyzing the data (post-hoc) can lead to bias. It’s best to select covariates before starting the analysis based on theoretical knowledge or preliminary testing.
Applications of Covariates in Different Statistical Analyses
- Regression Analysis
Covariates are frequently included in regression models (linear, logistic, etc.) to adjust for variables that may impact the relationship between the independent and dependent variables. In linear regression, covariates are added as additional predictor variables, while in logistic regression, they help improve the accuracy of predicting categorical outcomes.
- Analysis of Covariance (ANCOVA)
ANCOVA is a blend of ANOVA and regression. It allows for comparing means between groups while controlling for one or more covariates. This approach helps reduce variability due to covariates, leading to a more accurate comparison of group effects.
- Survival Analysis
In survival analysis, covariates are used to model the time until an event occurs (e.g., time until disease recurrence). Covariates such as age, treatment type, and baseline health can significantly affect survival outcomes, and including them helps improve the predictive power of the model.
- Propensity Score Matching
In observational studies, covariates can be used to create propensity scores, which represent the probability of receiving a treatment based on certain characteristics. Matching subjects with similar propensity scores helps reduce confounding and approximate the conditions of a randomized controlled trial.
- Multilevel Modeling
In hierarchical or multilevel models, covariates can be used at different levels of analysis. For example, in educational research, covariates might include both student-level factors (e.g., socioeconomic status) and school-level factors (e.g., funding level) to better understand individual and group-level effects on academic outcomes.
Potential Challenges and Considerations When Using Covariates
- Multicollinearity
When covariates are highly correlated with each other, multicollinearity can arise, making it difficult to interpret their individual effects. Techniques like variance inflation factor (VIF) analysis can help detect and address multicollinearity.
- Confounding Bias
Not all covariates will improve model accuracy. Including irrelevant covariates can introduce confounding bias and distort relationships within the model.
- Missing Data
In real-world data, covariates often have missing values. Imputation techniques, such as mean imputation or multiple imputation, are commonly used to handle missing data, but careful consideration is needed to ensure these methods don’t introduce additional bias.
- Interaction Effects
Sometimes, covariates interact with each other or with the main predictor variable, creating interaction effects. Identifying and including these interactions in the model can lead to a more nuanced understanding of the data but also increases model complexity.
Examples of Covariates in Research Studies
- Health Research: In studies assessing the impact of a new drug on recovery rates, age, pre-existing conditions, and baseline health status could all be covariates that influence recovery outcomes.
- Education Studies: Research on educational interventions often includes covariates like socioeconomic status, prior academic performance, and parental involvement to account for factors affecting student outcomes.
- Marketing Analysis: In examining the effectiveness of a marketing campaign, covariates might include customer age, income, previous purchase history, and geographical location.
- Environmental Studies: Research on pollution’s effects on health outcomes might use covariates such as proximity to industrial areas, individual smoking status, and socioeconomic factors.
Conclusion
Covariates play a pivotal role in statistical modeling, helping to control for confounding factors, increase precision, and improve the validity of research findings. Properly identifying and incorporating relevant covariates allows researchers to isolate the effects of the primary variables of interest, leading to more accurate and reliable conclusions. Whether in regression analysis, ANCOVA, survival analysis, or multilevel modeling, covariates enable researchers to explore complex relationships with greater confidence, providing a clearer picture of the factors at play in any study.
By understanding covariates and their applications, researchers and analysts can enhance their statistical models, avoid potential pitfalls, and produce insights that are both credible and actionable. The effective use of covariates represents an essential skill for statisticians, data scientists, and researchers across diverse fields, ultimately contributing to higher-quality research and better-informed decisions.

Clement Weber
Editor and Writer
Clement Weber is a talented writer and editor with a passion for drafting compelling narratives and refining content to perfection. With years of experience in both creative and professional writing, Clement has honed his skills in storytelling, copy editing, and content strategy. His expertise spans diverse genres, from fiction and feature articles to technical documentation and digital content. Known for his meticulous attention to detail and innovative ideas, Clement is dedicated to helping individuals and businesses effectively communicate their messages. When he's not immersed in words, he enjoys exploring literature, traveling, and discovering new cultures for inspiration.actful content. With expertise in both creative and professional writing, Cate excels at turning ideas into polished narratives that resonate with readers. Known for her meticulous editing skills and creative flair, Cate has worked on a wide range of projects, from manuscripts and academic papers to blogs and marketing materials. She is dedicated to helping clients achieve their vision with precision, clarity, and style.